INTRODUCTION
Weeds are recognized as the primary pests hindering crop establishment and growth, resulting in varying yield losses depending on the crop type (Gharde et al. 2018), constituting the principal constraint in crop production (Orke 2006), causing an average yield loss of 28% (Vilà et al. 2021). Therefore, weed management is critical for ensuring food security and environmental sustainability (Yaduraju & Rao 2013). The emergence of weed science as a distinct discipline is relatively recent, dating back less than 100 years, compared to other disciplines, such as plant pathology (Timmons 2005). The evolution of the weed science discipline stemmed from the discovery of the first synthetic herbicide, 2,4-dichlorophenoxyacetic acid (2,4-D), in 1941 by Pokorny, which subsequently underscored the importance of weed management.
Tillage and herbicides stand as the primary methods for weed removal from farms (MacLaren et al. 2020). However, the overwhelming success of herbicides has shaped the weed science discipline into a “herbicide-centered” discipline. This shift not only poses challenges for diversifying research in weed management but also imposes several concerns related to the environment, such as environmental hazards, contamination of natural resources, and the emergence of herbicide-resistant weeds, as well as consumer health. Herbicide residue contaminations are detected in soil as well as in surface and groundwater (Allinson et al. 2017; Sun et al. 2017). Another major concern associated with “herbicide-centered” weed management is food safety. Numerous studies have highlighted herbicide residues in fruits and vegetables, raising apprehensions among importing countries and consumers (Amjad et al. 2013; Matt et al. 2013). Kim et al. (2017) revealed the existence of various direct and indirect routes of chemical exposure. Therefore, the persistent use of herbicides is also an alarming concern for toxic exposure to humans and other non-target organisms.
These environmental and health-related challenges underscore the need for a broader perspective in weed management beyond a reliance on herbicides, such as a paradigm shift towards vegetation management on farms by understanding weed ecology, biology, and genetics (Chauhan et al. 2017; Clements et al. 2014). Furthermore, several aspects of weeds and their interactions remain poorly understood (Jordan et al. 2016). For example, the effects of fertilization on weed growth and development remain elusive (Little et al. 2021). Fertilizers act as a selection pressure in the competitive dynamics between crops and weeds. Therefore, strategic weed management is crucial to ensure crops outcompete weeds. Desired timing for weed control is when weeds and crop are still in symmetrical competition level, and not in asymmetrical competition where weeds outcompete crops (Little et al. 2021). Despite the urgency for effective weed management, the lack of detailed information on weed biology has resulted in weak predictions of weed species on farms; specifically, the “how” and “why” aspects have not been fully explored (Ward et al. 2014). These knowledge gaps limit the science-based strategies in the application of weed management practices, particularly in developing countries (Chaney & Baucom 2012; Chauhan et al. 2017).
Several weed biology articles specifically have been published in Canada and Australia since the 1970s (Cavers et al. 2013; Groves & Panetta 2014); however, the species information is limited to comprehensive literature reviews, offering limited new quantitative information (Ward et al. 2014). Additionally, understanding the growth and development of weed species can serve as a basis for integrating different weed control methods (Chaney & Baucom 2012). The parameters determining plant growth include plant height, stem thickness, and biomass, whereas parameters such as the number of leaves and number of inflorescences determine plant development (Dambreville et al. 2015).
The Amaranthaceae family consists of 70 species, of which a few are vegetables and some are weeds. Slender amaranth (Amaranthus viridis L.) is widely distributed, being native to 33 countries and an introduced species in 98 countries (POWO 2022). Amaranthus viridis is an annual broadleaf weed with a C4 photosynthetic pathway and is found on farms and in open habitats, such as roadsides. The weed species utilizes seed propagation mechanisms. Amaranthus viridis has the second highest mean field density and high relative abundance among other weed species found in smallholder maize farms in Malim Nawar, Malaysia, per field surveys conducted in 2017, 2018, and 2020 (Tong & Lim 2022). The high mean density and relative abundance of this weed species reflect the degree of difficulty in its control (de Mol et al. 2015).
This study aimed to investigate the functional relationships between traits (number of leaves, number of inflorescences, and plant height) of A. viridis in wild populations and their growth responses to NPK 15:15:15 and NPK 12:12:17. The present study demonstrates how enhanced knowledge of weed biology could lead to practical advances benefiting farmers; thus, our study will provide a foundation to initiate the development of science-based weed management technologies.
MATERIALS AND METHODS
Experimental design
The present study evaluated wild A viridis. A total of 30 seedlings (5–20 cm high) from a single location in Kampar, Malaysia was used to ensure similar batch of seeds (Hanzawa & Kalisz 1993; Kirkpatrick 1984). The seedlings were transferred to polybags (20 cm × 15 cm × 30 cm; L × W × H) filled with soil from the site from where A. viridis were obtained, with one seedling planted in a polybag. The soils used were tailing parent material from the site where seedlings were found, which was a pale brown to brown upper layer of 30–70 cm. The soil composition included sandy clay to clay, weak fine subangular blocky, friable texture, overlaying brown silty clay to clay, olive-brown to brown coarse sandy clay loam with some clay balls.
Seedlings were planted in a Randomized Complete Block Design (RCBD) with six blocks for five treatments in a shade house, maintained at a relative light intensity of 50% in the north-south direction. Each treatment comprised six replicates. The five treatments included a control without fertilization and four sets fertilized with different dosages (1, 2, 4, and 8 g per polybag) of Behn Meyer Nitrophoska® Green NPK 15-15-15+2S, produced by Eurochem Agro (the manufacturer’s details are not known). Fertilizer was applied one month after transplanting and subsequently on a weekly basis throughout the study period from March to September 2020. Additionally, six plants in the wild population were studied without any treatment and were not transplanted. The number of leaves, number of inflorescences, and height (cm) of each plant were recorded weekly. Plant organs were considered to reflect the functional characteristics of growth, competitive ability, and reproduction (Garnier & Navas 2012). The experiment was repeated from May to September 2021 for five months with another set of 30 plants. The fertilizer was changed to AgroBridge NPKMg 12-12-17-2+TE, a muriate of potash (MOP) based fertilizer imported from Europe (the manufacturer’s details are not known). The duration of both experiments was similar to the maize planting cycle or longer to understand plant responses to fertilizer treatments. These fertilizers were chosen based on their common use by smallholders in maize planting, as reported in weed surveys conducted by Tong & Lim (2022).
Statistical analyses
The Shapiro–Wilk normality and Q-Q plot tests were performed to determine whether a variable had a normal distribution. The null hypothesis of normal population distributions was accepted when p > 0.05 and rejected otherwise. If the normality assumption was met, the Pearson correlation coefficient (r) and linear regression (R2) were assessed to analyze the relationship between the number of leaves, number of inflorescences, and plant height. Three models were analyzed to estimate the correlation between the traits: Model 1, where the number of leaves was used as the dependent variable and plant height as the independent variable; Model 2, where inflorescence was the dependent variable and the number of leaves served as the independent variable; and Model 3, where the number of inflorescences was the dependent variable and the number of leaves and plant height were the independent variables. The gradient (β) was tested for significance. A significant relationship was observed when the gradient was not zero (p < 0.001). SPSS version 20.0 was used for statistical analyses.
RESULTS AND DISCUSSION
The null hypothesis of population normality was retained for A. viridis in wild populations and under the fertilizer treatments NPK 15:15:15 and 12:12:17 when p > 0.05. These values were normally distributed.
Model 1: Dependent variable: number of leaves, independent variable: plant height
The number of leaves and plant height were significantly positively correlated (r = 0.679) for A. viridis under NPK 12:12:17 (b = 21.821, SEb = 5.900, β = 0.679, t = 3.698, p = 0.001; Figure 1A). The slope coefficient for the leaves was 21.82, suggesting that a 1 cm increase in plant height increases the number of leaves by 21.82. The R2 value was 0.461, indicating that 46.1% of the variation in the number of leaves could be explained by the model containing only plant height.
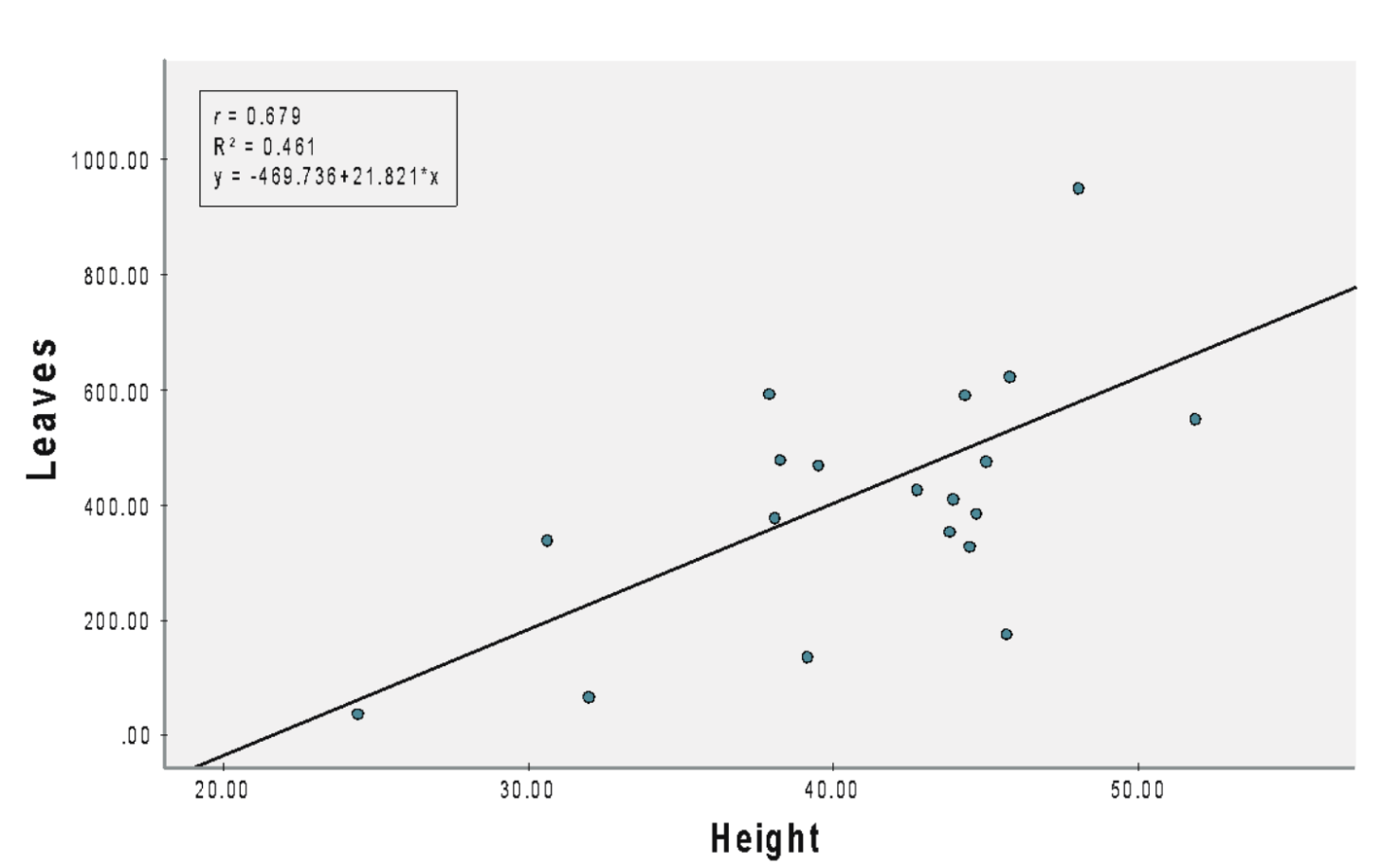
(A) NPK 12:12:17
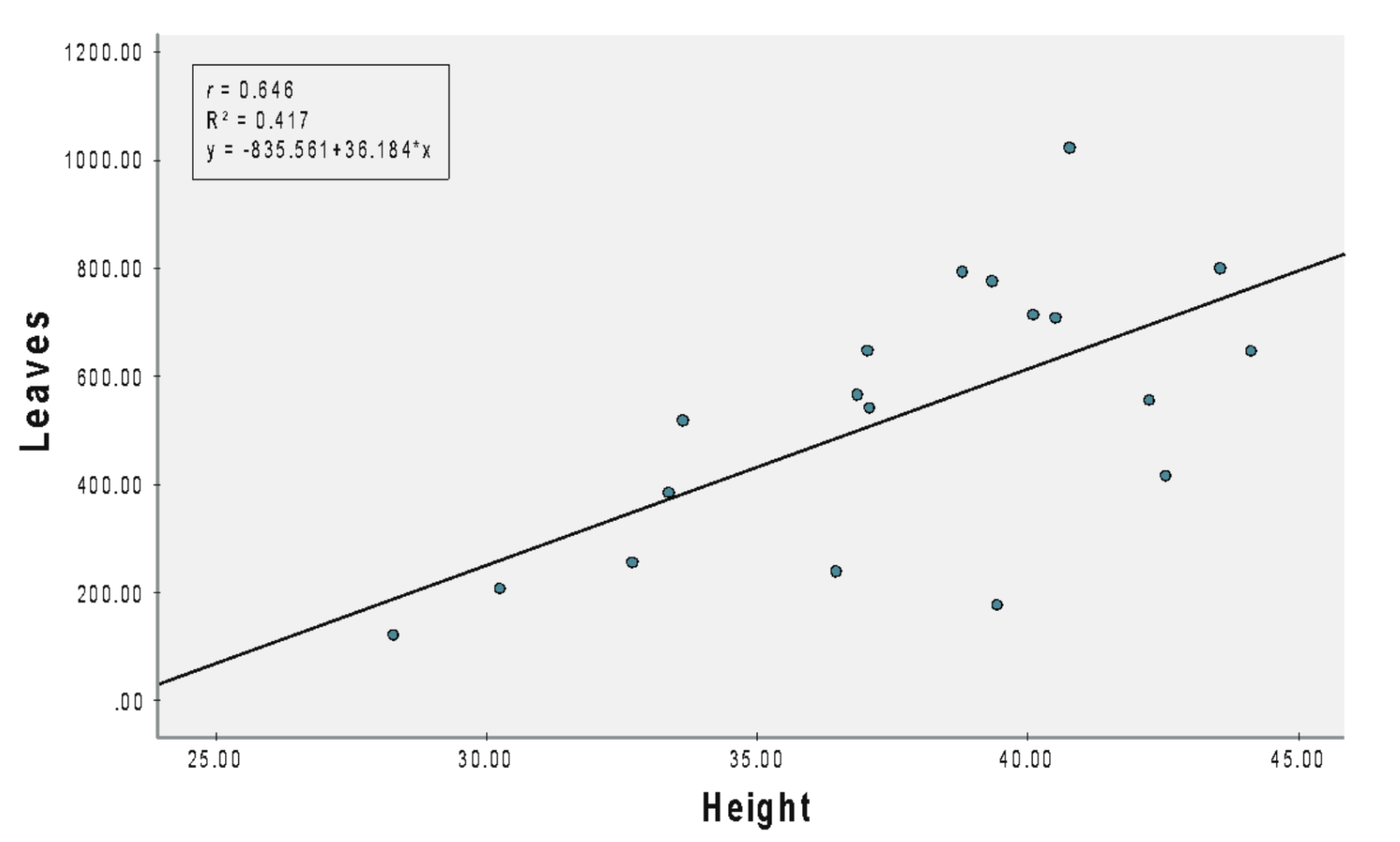
(B) NPK 15:15:15
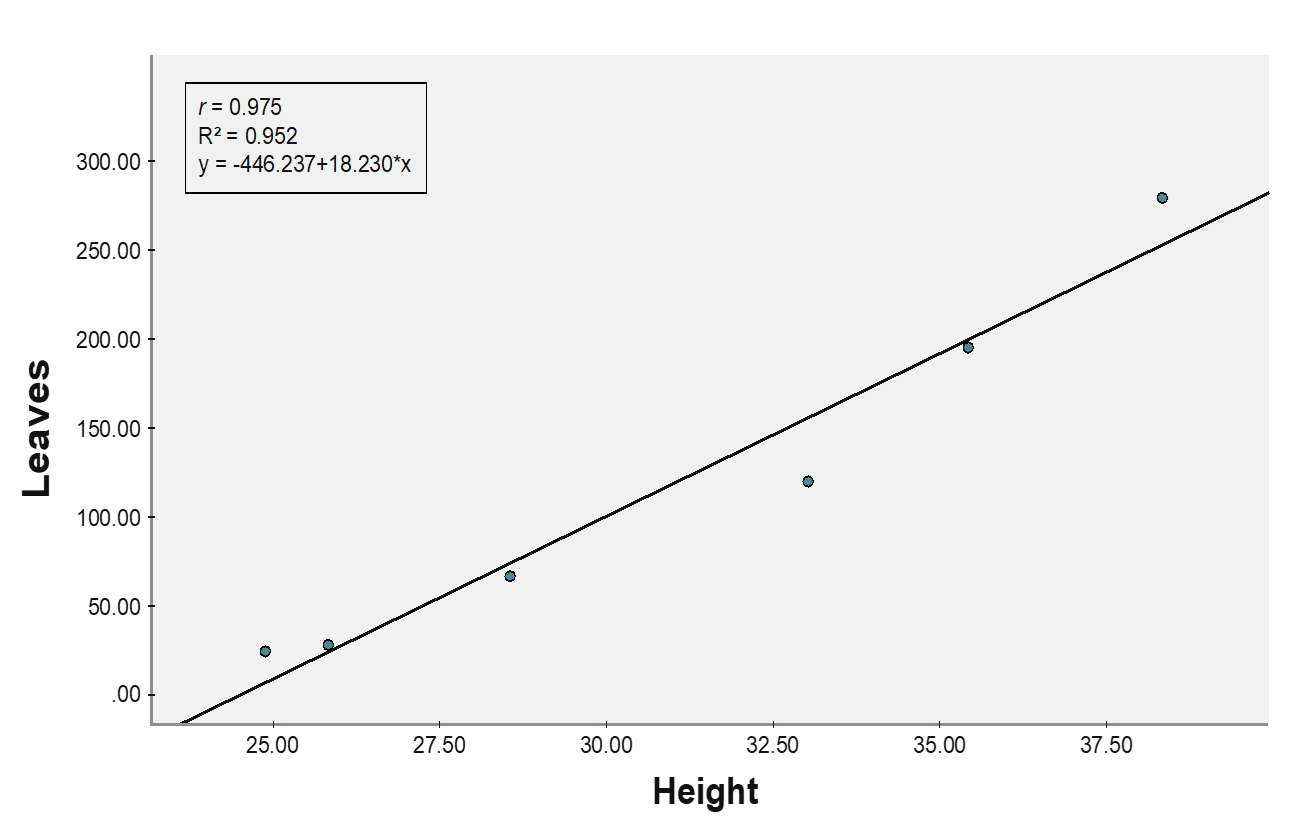
(C) Wild plants
Pearson’s correlation coefficient (r = 0.646) revealed a significant (b = 36.184, SEb = 10.383, β = 0.646, t = 3.485, p < 0.001) positive relationship between the number of leaves and plant height for A. viridis under NPK 15:15:15 (Figure 1B). An increase in height by 1 cm led to an increment of 36.18 leaves, and R2 suggested that 41.7% of the variation in the number of leaves was accounted for by height.
Concordantly, a positive relationship between plants and the number of leaves (r = 0.975) was observed for the wild population (Figure 1C). Plant height significantly predicted the number of leaves (b = 18.422, SEb = 2.015, β = 0.975, t = 8.864, p = 0.001). The model explained 95.2% of the variance in the number of leaves, and a 1 cm in height increment speculated to increase the number of leaves by 18.23.
Model 2: Dependent variable: number of inflorescences, independent variable: number of leaves
Assessment using Model 2 revealed a positive correlation (r = 0.888) between the numbers of inflorescences and leaves for A. viridis under fertilizer NPK 12:12:17 (Figure 2A). Linear regression analysis revealed a significant relationship (b = 0.270, SEb = 0.035, β = 0.888, t = 7.706, p < 0.001). The slope coefficient for the leaves was 0.270, which indicated an increase in the number of inflorescences by 0.270 for each leaf. The model revealed that leaves accounted for 78.8% (R2 = 0.788) of the variance in inflorescences.
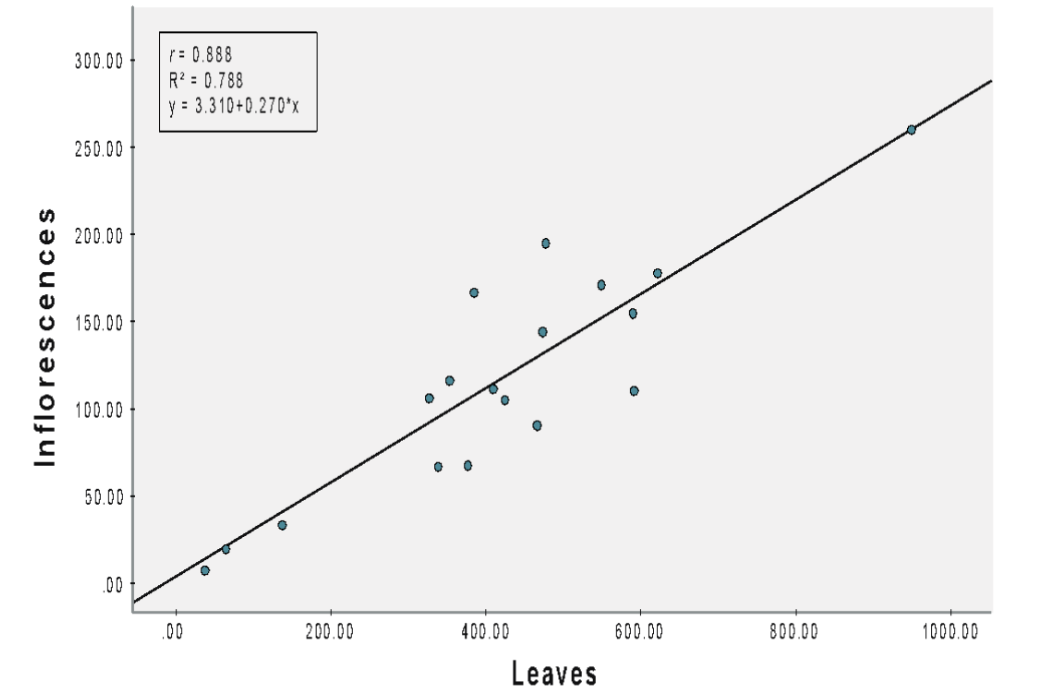
(A) NPK 12:12:17
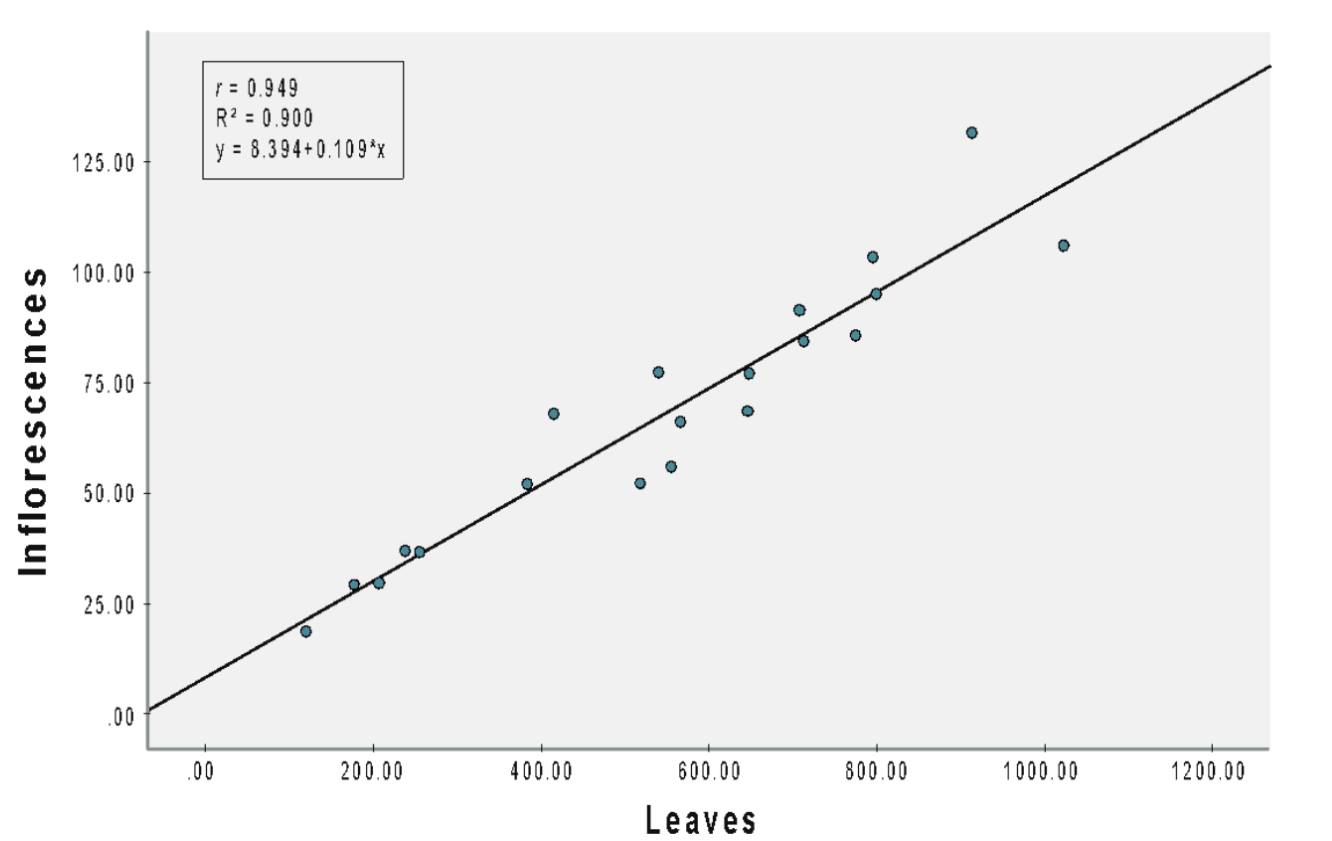
(B) NPK 15:15:15
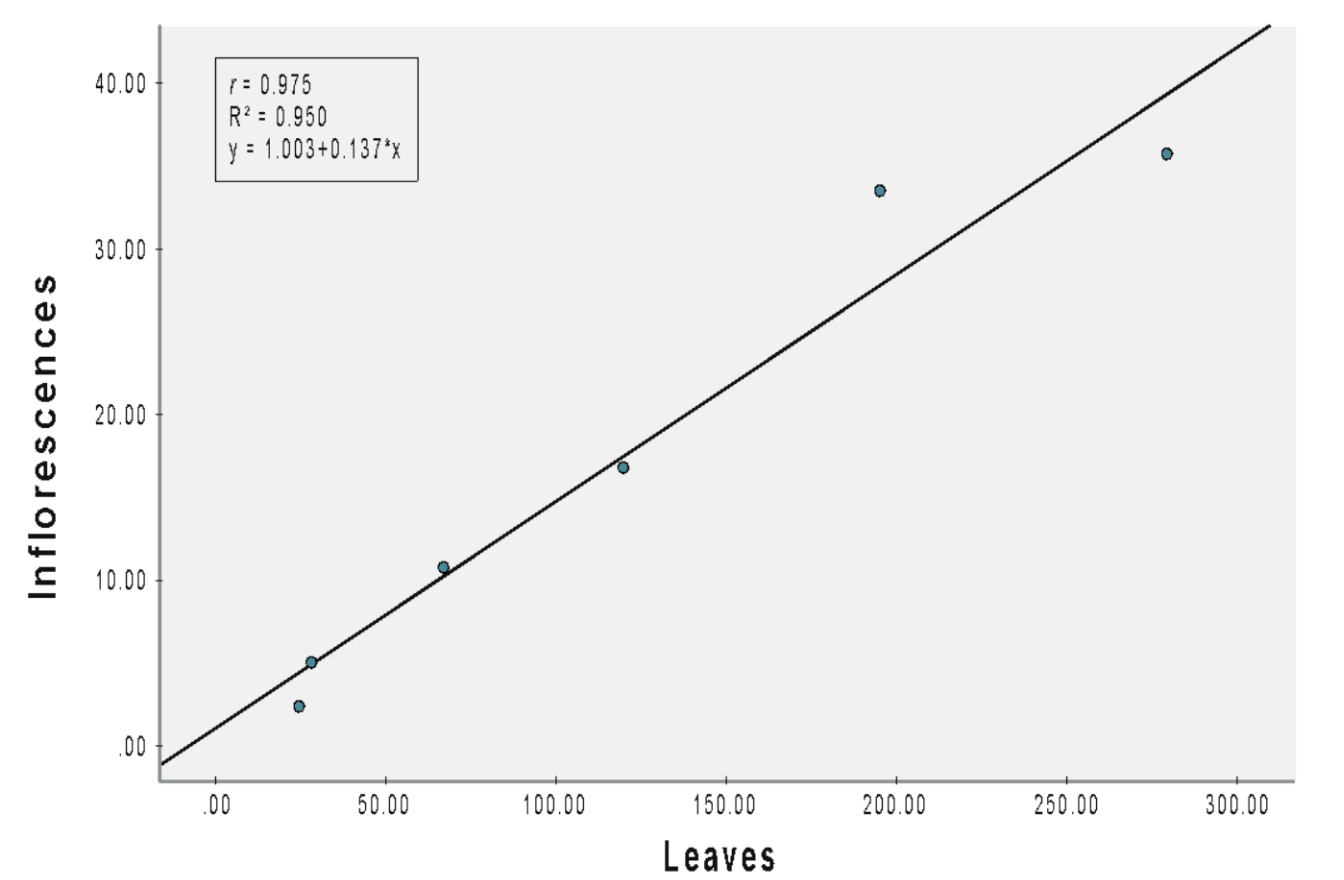
(C) Wild plants
For treatments under NPK 15:15:15, a significant (b = 0.109, SEb = 0.009, β = 0.949, t = 12.708, p < 0.001) positive correlation (r = 0.949) was observed (Figure 2B). The slope coefficient for inflorescences was 0.109, signifying that the number of inflorescences increased by 0.109 for each additional leaf. The R2 value indicated that 90% of the variation in inflorescences could be explained by the number of leaves.
The correlation coefficient (r = 0.975) between leaves and inflorescences in wild plants was robust and statistically significant (b = 1.003, SEb = 0.016, β = 0.975, t = 8.745, p = 0.001; Figure 2C). Specifically, each additional leaf corresponds to a notable increase of 0.137 inflorescences. The proportion of variance in the number of inflorescences explained by the number of leaves was 95%.
Model 3: Dependent variable: number of inflorescences, independent variable: number of leaves and plant height
The scatterplot revealed a strong linear relationship between the number of inflorescences and leaves and height combined, with an r-value of 0.911 (Figure 3A), which was statistically significant [b = 0.212 (leaves), 2.763 (height), SEb = 0.044 (leaves), 1.415 (height), β = 0.696 (leaves), 0.282 (height), t = 4.810 (leaves), 1.952 (height), p < 0.001]. Specifically, each inflorescence is associated with an increase of 0.212 leaves and 2.763 cm in height. Furthermore, the combined effect of leaves and height accounts for 83.1% of the variance in inflorescences.
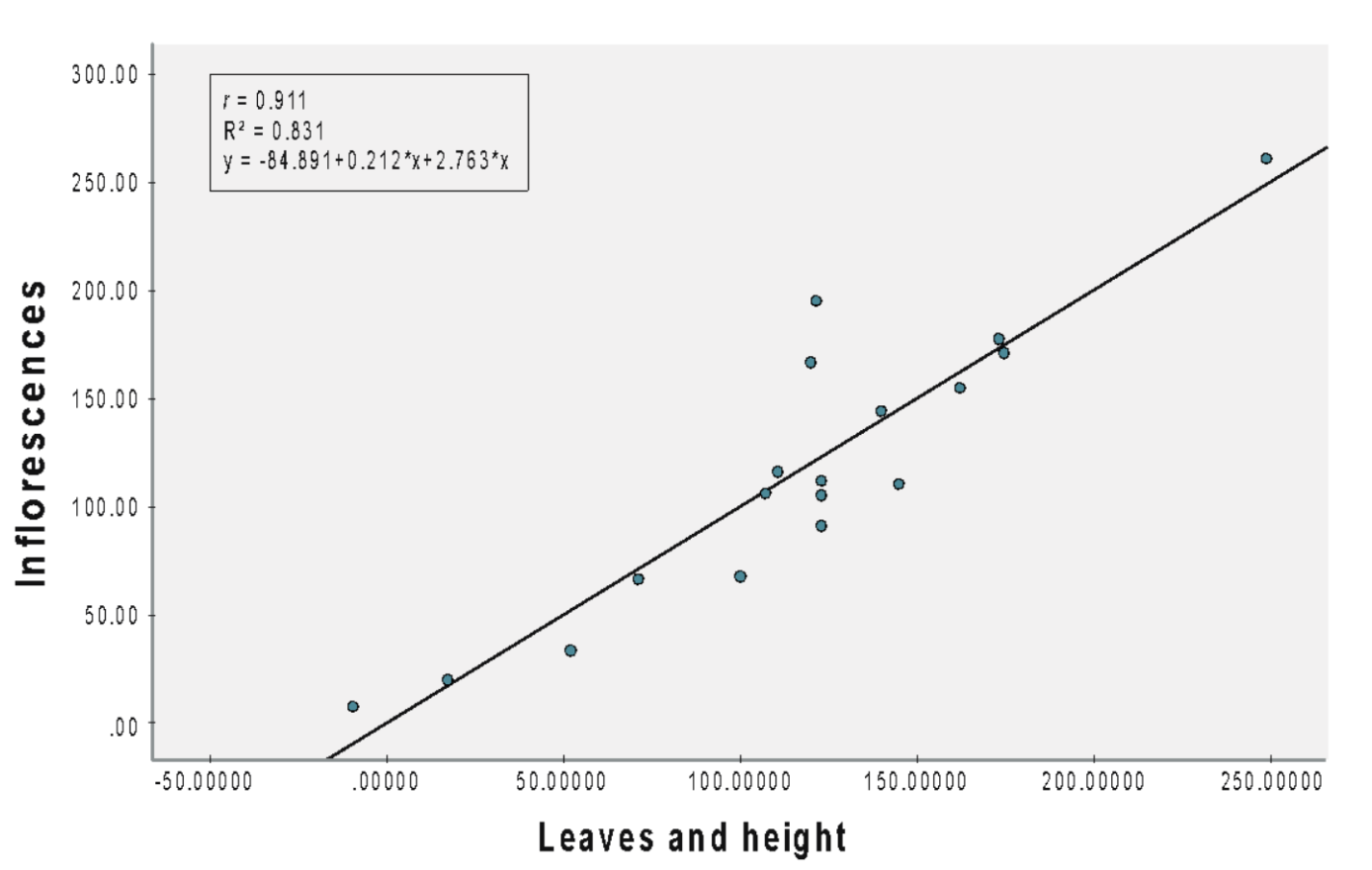
(A) NPK 12:12:17
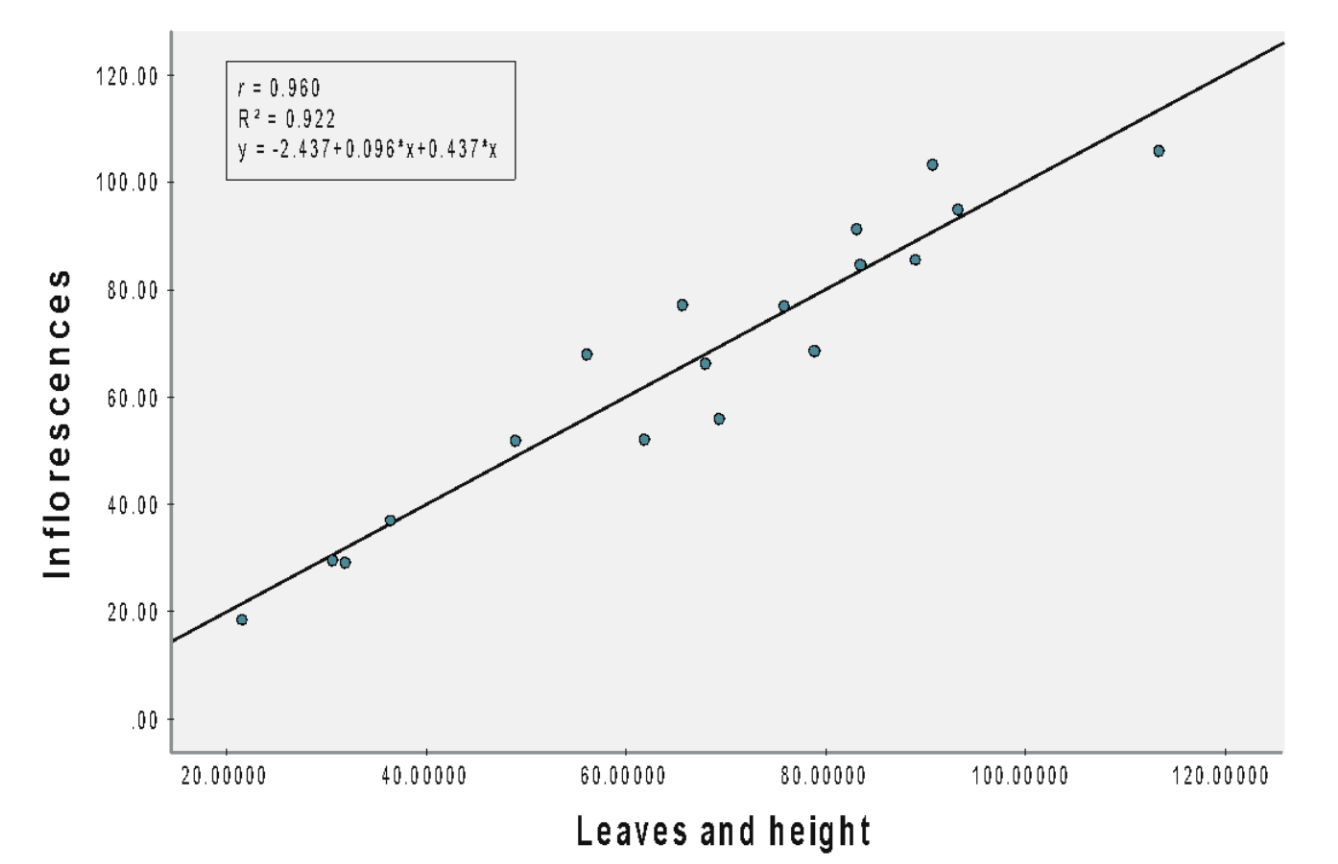
(B) NPK 15:15:15
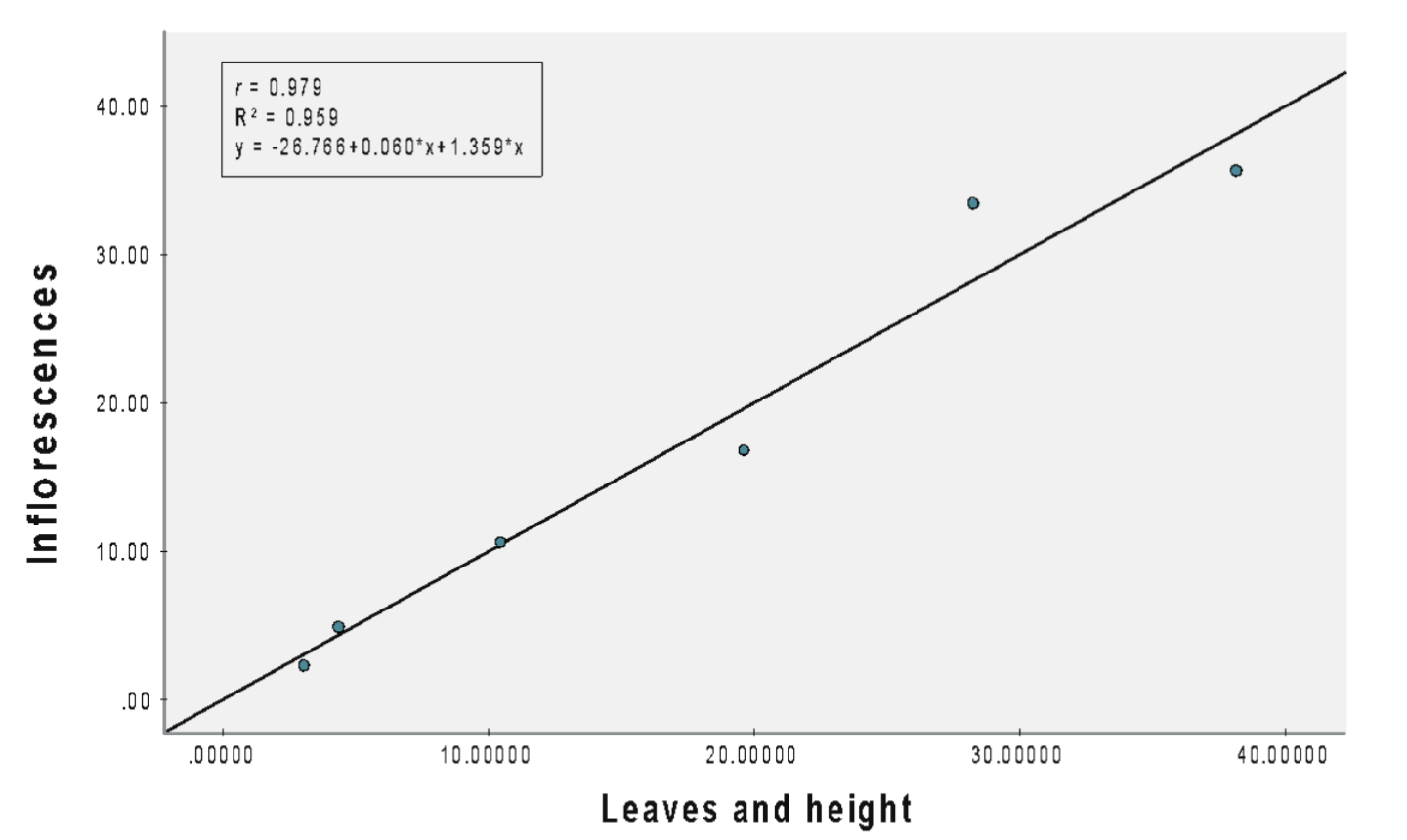
(C) Wild plants
The Pearson correlation coefficient of 0.960 indicated a strong linear relationship between inflorescence and both height and the number of leaves (Figure 3B), with a significant relationship (b = 0.096 (leaves), 0.437 (height), SEb = 0.010(leaves), 0.540 (height), β = 0.910 (leaves), 0.074 (height), t = 9.935 (leaves), 0.809 (height), p < 0.001). For each inflorescence, the number of leaves was increased by 0.096 and the height by 0.437 cm. Both leaves and height collectively explained 92.2% of the variation in the number of inflorescences.
Additionally, a robust correlation of 0.979 was observed between inflorescences and both leaves and height (Figure 3C). Our findings affirmed that the number of leaves and plant height significantly predicted the number of inflorescences [b = 0.078 (leaves), 1.359 (height), SEb = 0.085 (leaves), 1.650 (leaves), β = 0.449 (leaves), 0.557 (height), t = 0.820 (leaves), 1.609 (height), p = 0.008], with 95.9% of the variance in the number of inflorescences was accounted for by leaves and height.
Plant height is an indicator of organ growth, growth rate, and leaf number (Dambreville et al. 2015; Kunstler et al. 2016) and indicates reproductive maturity in herbaceous plants (Garnier & Navas 2012). Members of Amaranthus can grow up to a maximum height of 2.2 m (Martínez-Núñez et al. 2019). Concordant with our findings, those of previous studies have demonstrated that A. retroflexus shows a linear increase in height throughout its life cycle (Li et al. 2015; Little et al. 2021). Leaves play a crucial role in resource acquisition, while inflorescences are pivotal for reproduction. A higher leaf number indicates better growth rates and exhibits a strong correlation with other plant traits (He et al. 2020). The number of inflorescences is a reliable indicator for plants using seed production strategies, which facilitates the prediction of seed numbers (Chaney & Baucom 2012). Each inflorescence of A. viridis contained an average of 347 seeds, with a seed length of 1.25 ± 0.15 mm.
Growth and developmental parameters exhibit interconnectedness throughout the plant life cycle (Dambreville et al. 2015; He et al. 2020). The scatterplots generated using Models 1, 2, and 3 revealed strong positive relationships between the dependent and independent variables. The independent variables in these three models significantly predicted the dependent variables, with the latter demonstrating an increase corresponding to the rise in the independent variable(s). Moreover, weeds in wild populations exhibited growth and development patterns mirroring those of weeds studied under NPK 15:15:15 and NPK 12:12:17. This finding contradicts the hypothesis of Lavorel and Garnier (2012), which posited distinct trait responses for wild plants and farm weeds. Wild and arable weeds largely share ecological strategies, including reproduction (Bourgeois et al. 2019). Previous studies have shown that trait-based approaches can overcome the challenges posed by the heterogeneity within and between weed species (Gaba et al. 2017; Lavorel & Garnier 2002). In this study, the analysis of three models aimed to explore the functional relationships between traits revealed that the findings could guide the selection of the model for a more comprehensive understanding and accurate prediction of other weed species while considering data availability for certain traits.
Fertilizers exert the primary selection pressure on arable weeds. The positive correlations observed in this study indicate the co-optimization of A. viridis. Under favorable conditions, such as nutrient abundance, weeds strategically enhance both vegetative and reproductive aspects, consequently increasing plant fitness (Li et al. 2015; Little et al. 2021). An increase in vegetative components, such as height and leaves, promotes the competitive ability of weed species (Hegazy et al. 2005). This study discerned that continuous resource availability promotes co-optimization between traits. Weeds continue to grow and produce inflorescences until senescence (Hegazy et al. 2005). The response-effect framework can clarify patterns that reflect plant functional responses and aid in grouping species at the community level, such as facilitating the upscaling of individual plant responses to fertilizers (Lavorel & Garnier 2012). The hub trait (i.e., inflorescence) interacts with other traits and has a higher degree of correlation, whereas the mediator trait indicates the betweenness of the two traits which is a single spectrum of traits (He, et al., 2020), suggesting Model 3 was a hub trait, and Models 1 and 2 were mediator traits.
In this study, an assessment of these traits on farms provided insights into aboveground growth and development patterns. Analyzing the growth development patterns in individual plant behavior at the trait level represents the initial phase for species comparison and broader generalization, marking the commencement of a systemic approach to weed control in practice (Little et al. 2021). Weeds undergo evolutionary adaptations within the temporal and spatial dimensions of the farm environment. To gain a comprehensive understanding, research efforts could be expanded to include other weed species within the same farm, investigating whether their fundamental biology aligns with that of A. viridis or similar species from different locations. Effective weed management practices can be implemented by acquiring detailed information on the growth and development parameters of weed species on farms. Moreover, the review of post-emergence herbicide application, encompassing considerations such as dosage, timing, and frequency at specific heights to minimize seed numbers, could be an integral component of vegetation management on farms.
Biological-intensification weed management involving holistic and sustainable approaches is complex and requires careful consideration and handling of several factors (Gaba et al. 2017; Little et al. 2021). Research efforts using this framework should actively engage smallholders in validation processes and joint efforts with smallholders to review their herbicide applications are essential. To conclusively demonstrate growth and development patterns, a follow-up program combining trait and response-and-effect approaches is necessary (Gaba et al. 2017; Garnier & Navas 2012). Experimental studies have suggested a timeframe of four to six years in the fields, taking into account the pressures the smallholder farmers face from weeds, which significantly influence their decisions and practices (Gaba et al. 2016). Therefore, facilitating the understanding of the smallholders regarding the basic concepts and appropriate technologies through continuous learning is crucial (Terlau et al. 2019). Furthermore, research on farmer participation is pragmatic for the development of sustainable weed management (Hall et al. 2000). Although chemical herbicides remain a central control method, refining their use by adjusting the dosage and application timing of fertilizers can contribute to reducing overall herbicide usage (Bastiaans et al. 2000).
LIMITATIONS
This study has some limitations in its examination of the germination rate, vegetative stage from germination to the emergence of the first inflorescence, and overall life span from germination to senescence under different environmental conditions (i.e., wild populations, NPK 15:15:15, and NPK 12:12:17). To ensure practical feasibility, the growth and development of A. viridis treated with fertilizers under farm conditions were not compared. Furthermore, this study focused on a single species with a single-density approach instead of investigating intra- and inter-specific weed competition. Another challenge in studying weeds is their plasticity within specific habitats and at different densities.
CONCLUSION
This study demonstrated the patterns of functional attributes of A. viridis in response to fertilizers and wild populations. Traits such as the number of leaves, number of inflorescences, and plant height explained individual plant responses. Models 1, 2, and 3, focusing on the interactions among these traits, revealed significant r and R2. Specifically, Model 3 of the inflorescence to leaf numbers and height showed higher r and R2 values. This study predicted that A. viridis would respond to selection pressures such as fertilizers. These three models serve as valuable tools to predict the dependent variables based on the types of available data. The applicability of these models could be extended to broadleaved species with seeds as the primary reproduction mechanism, offering insights into how the traits of a weed species in the wild and responses to fertilizer contribute to its inherent growth and responses. The findings of this study hold potential for broader application, allowing the exploration of these mechanisms in other species for sustainable weed management with the involvement of smallholders. Overall, this study demonstrates how enhanced knowledge of weed biology and responses to selection pressures such as fertilizers could lead to practical advances benefiting farmers. Nevertheless, future research should delve into weed–crop interactions within agricultural settings and optimal fertilization times by integrating the findings of this study to develop a science-based weed management strategy.
ACKNOWLEDGMENTS
Authors state no funding involved. PST was responsible for conceptualization, methodology and data analysis. KNC, JZT and HYY were responsible for data collection. All authors contributed to manuscript writing and approved the final version of the manuscript.
REFERENCES
- Allinson M, Zhang P, Bui A, Myers JH, Pettigrove V, Rose G, Salzman SA, Walters R, Allinson G. 2017. Herbicides and trace metals in urban waters in Melbourne, Australia (2011-12): concentrations and potential impact. Environ Sci Pollut Res 24(8):7274-84. doi: 10.1007/s11356-017-8395-9
- Amjad M, Ahmad T, Iqbal Q, Nawaz A., Jahangir MM. 2013. Herbicide contamination in carrot growth in Punjab, Pakistan. Pak J Agric Sci 50(1): 7-10.
- Badri M, Lubis DP, Susanto D, Suharjito D. 2018. Sistem komunikasi peringatan dini pencegahan kebakaran hutan dan lahan di Provinsi Riau [Early warning communication system for preventing forest and land fires in Riau Province]. Jurnal PIKOM (Penelitian Komunikasi Dan Pembangunan), 19(1), 1-16.
- Bastiaans L, Kropff MJ, Goudriaan J, van Laar HH. 2000. Design of weed management systems with a reduced reliance on herbicide poses new challenges and prerequisites for modelling crop-weed interactions. Field Crops Res 67(2): 161-79.
- Bourgeois B, Munoz F, Fried G, Mahaut L, Armengot L, Denelle P, Storkey J, Gaba S, and Violle C. 2019. What makes a weed a weed? A large-scale evaluation of arable weeds through a functional lens. Am J of Bot 106(1):90-100. doi: 10.1002/ajb2.1213
- Cavers PB, Darbyshire SJ, Mulligan, GA. 2013. History of the series on the biology of Canadian weeds. Can J Plant Sci 93: 351-61.
- Chaney L, Baucom RS. 2012. The evolutionary potential of Baker’s weediness traits in the common morning glory, Ipomea purpurea (Convolvulaceae). Am J of Bot 99(9):1524-30.
- Chauhan BS, Matloob A, Mahajan G, Aslam F, Florentine SK, Jha P. 2017. Emerging challenges and opportunities for education and research in weed science. Front Plan Sci [Internet]. [cited 2023 Mar 20]; 8:1537. doi: 10.3389/fpls.2017.01537
- Clements DR, DiTommasa A, Hyvonen T. 2014. Ecology and management of weeds in a changing climate. In: Chauhan BS, Mahajan G, editors. Recent advances in weed management: Springer Publishing. p. 13-38.
- Dambreville A, Lauri P-É, Normand F, Guédon Y. 2015. Analysing growth and development of plants jointly using developmental growth stages. Ann Bot 115(1): 93-105.
- de Mol, F., von Redwitz, C. and Gerowitt, B., 2015. Weed species composition of maize fields in Germany is influenced by site and crop sequence. Weed Research, 55(6): 574-585.
- Fryer JD. 1981. Weed management: fact or fable? Philosophical Transactions of the Royal Society of London. Proc Royal Soc B 295: 185-97.
- Gaba S, Gabriel E, Chadoeuf J, Bonneu F, Bretagnolle V. 2016. Herbicides do not ensure for higher wheat yield, but eliminate rare plant species. Sci Rep [Internet]. [cited 2023 Mar 21]; 6. doi: 10.1038/srep30112
- Gaba S, Perronne R, Fried G, Gardarin A, Bretagnolle F, Biju-Duval L, Colbach N, Cordeau S, Fernandez-Aparicio M, Gauvrit C, Gibot-Leclerc N, Guillemin J-P, Moreau D, Munier-Jolain N, Strbik F, Reboud X. 2017. Response and effect traits of arable weeds in agro-ecosytems: a review of current knowledge. Weed Res. 57(3): 123-47.
- Garnier E, Navas M-L. 2012. A trait-based approach to comparative functional plant ecology: concepts, methods and applications for agroecology. A review. Agron for Sustain Dev 32:365-99. doi: 10.1007%2Fs13593-011-0036-y
- Gharde Y, Singh PK., Dubey RP, Gupta, PK. 2018. Assessment of yield and economic losses in agriculture due to weeds in India. Crop Protection 107:12-8. doi: 10.1016/j.cropro.2018.01.007
- Groves RH, Panetta FD. 2014. The biology of Australian weeds – a short history of the series. Plant Prot Q 29(4): 127-30.
- Hall JC, Van Eerd LL, Miller SD, Owen MDK, Prather TS, Shaner DL, Singh M, Vaughn K, Weller, SC. 2000. Future research directions for weed science. Weed Technol [Internet]. [cited 2023 Mar 21]; 14(3):647-58. Available from: doi: 10.1614/0890-037X(2000)014[0647:FRDFWS]2.0.CO;2
- Hanzawa FM, Kalisz S. 1993. The relationship between age, size, and reproduction in Trillium grandiflorum (Liliaceae). Am J Bot 80(4): 405-10. doi: 10.2307/ 2445387
- He N, Li Y, Liu C, Xu L, Li M, Zhang J, He J, Tang Z, Han X, Ye Q, Xiao C, Yu Q, Liu S, Niu S, Li S, Sack L, Yu G. 2020. Plant trait networks: improved resolution of the dimensionality of adaptation. Trends Ecol Evol 35(10):908-18. doi: 10.1016/j.tree.2020.06.003
- Hegazy AK, Fahmy GM, Ali M, Gomaa NH. 2005. Growth and phenology of eight common weed species. J Arid Environ 61:171-83. doi: 10.1016/j.jaridenv.2004.07.005
- Jordan N, Schut M, Graham S, Barney JN, Childs DZ, Christensen S, Cousens RD, Davis AS, Eizenberg H, Ervin DE, Fernandez-Quintanilla C, Harrison LJ, Harsch MA, Heijting S, Liebman M, Loddo D, Mirsky SB, Riemens M, Peltzer DA, Renton M, Williams M, Recasens J, Sønderskov M. 2016. Transdisciplinary weed research: new leverage on challenging weed problems? Weed Res 56(5): 345-58.
- Kim K-H, Kabir E, Jahan SA. 2017. Exposure to pesticides and associated human health effects. Sci Total Environ 575(1):525-35. doi: 10.1016/j.scitotenv.2016.09.009
- Kirkpatrick M. 1984. Demographic models based on size, not age, or organisms with indeterminate growth. Ecol 65(6): 1874-84. doi: 10.2307/1937785
- Kunstler G, Falster D, Goomes DA, Hui F, Kooyman RM, Laughlin DC, Poorter L, Vanderwel M, Vielledent G, Wright SJ, Aiba M, Baraloto C, Caspersen J, Cornelissen JHC, Gourlet-Fleury S, Hanewinkel M, Herault B, Kattge J, Kurokawa H, Onoda Y, Peñuelas J, Poorter H, Uriarte M, Richardson S, Ruiz-Benito P, Sun I-F, Ståhl G, Swenson NG, Thompson J, Westerlund B, Wirth C, Zavala MA, Zeng H, Zimmermen JK, Zimmermen NE, Westoby M. 2016. Plant functional traits have globally consistent effects on competition. Nature 259: 204-20. doi: 10.1038/nature16476
- Lavorel S, Garnier E. 2002. Predicting changes in community composition and ecosystem functioning from plant traits: revisiting the Holy Grail. Funct Ecol 16: 545-56. doi: 10.1371/journal.pone.0127795
- Li H, Lindquist JL, Yang Y. 2015. Effects of sowing date on phenotypic plascticity of fitness-related traits in two annual weeds on the Songnen Plain of China. PLoS ONE [Internet]. [cited 2023 Mar 18]; 10(5), Available from: doi: 10.1371/ journal.pone.0127795
- Little, NG, DiTommaso A, Westbrook AS, Ketterings QM, Mohler CL. 2021. Effects of fertility amendments on weed growth and weed-crop competition: a review. Weed Sci 69: 132-46.
- MacLaren C, Storkey J, Menegat A, Metcalfe H, Dehnen-Schmutz K. 2020. An ecological future for weed science to sustain crop production and the environment. A review. Agron Sustain Dev [Internet]. [cited 2023 Mar 22]; 40(24). doi: 10.1007/s13593-020-00631-6
- Martínez-Núñez M, Ruiz-Rivas M, Vera-Hernández PF, Bernal-Muñoz R, Luna-Suárez S, Rosas-Cárdenas FF, 2019. The phenological growth stages of different amaranth species grown in restricted spaces based in BBCH code. S Afr J Bot [Internet]. [cited 2023 Mar 22]; 124:436-43. doi: 10.1016/ j.sajb.2019.05.035
- Matt D, Pehme S, Peetsmann E, Luik A, Meremäe K. 2013. Pesticide residues in Estonian local and imported food in 2008-2011. Acta Agric Scand B Soil Plant Sci 63(1): 78-84. doi: 10.1080/09064710.2013.793383
- Orke E-C. 2006. Crop losses to pests. J Agric Sci [Internet]. [cited 2023 Mar 23];144(1): 31-43. doi: 10.1017/S0021859605005708
- POWO [Internet]. 2022. Plants of the world online. Facilitated by the Royal Botanic Gardens, Kew. [cited 2023 Mar 20] Available from: https://powo.science.kew.org/taxon/urn:lsid:ipni.org:names:316349-2#distribution-map
- Sun JT, Pan LL, Zhan U, Tsang DCW, Zhu LZ, Li XD. 2017. Atrazine contamination in agricultural soils from the Yangtze River Delta of China and associated health risks. Environ Geochem Health 39(2): 369-78. doi: 10.1007/s10653-016-9853-x
- Terlau W, Hirsch D, Blanke M. 2019. Smallholder farmers as a backbone for the implementation of the Sustainable Development Goals. Sustain Dev 27: 523-9. doi: 10.1002/ sd.1907
- Timmons FI. 2005. A history of weed control in the United States and Canada. Weed Sci 53: 748-61. doi: 10.1017/S0043174500079807
- Tong PS, Lim, TM. 2022. Weed composition and maize yield in a former tin-mining area: a case study in Malim Nawar, Malaysia. Open Agric [Internet]. [cited 2023 Mar 20]; 7: 478-85. doi: 10.1515/opag-2022-0117
- Vilà M, Beaury EM, Blumenthal DM, Bradley BA, Early R, Laginhas BB, Trillo A, Dukes JS, Sorte CJB, Ibáñez I. 2021. Understanding the combined impacts of weeds and climate change on crops. Environ Res Lett [Internet]. [cited 2023 Mar 20]; 16. doi: 10.1088/1748-9326/abe14b
- Ward SM, Cousens RD, Bagavathiannan MV, Barney JN, Beckie HJ, Busi R, Davis AS, Dukes JS, Forcella F, Freckleton RP, Gallandt ER, Hall LM, Jasieniuk M, Lawton-Rauh A, Lehnhoff EA, Liebmann M, Maxwell BD, Mesgaran MB, Murray JV, Neve P, Nunez MA, Pauchard A, Queenborough SA, Webber BL. 2014. Agricultural weed research: a critique and two proposals. Weed Sci 62: 672-8.
- Yaduraju NT, Rao AN. 2013. Implications of weeds and weed management on food security and safety in the Asia-Pacific region. In: Bakar B, Kurniadie D, Tjitrosoedirdjo S, editors. 24th Proceedings: 2013 Oct 22-25; Bandung, Indonesia, Bandung, Indonesia: Weed Society of Indonesia & Padjadjaran University. p.13-30.